Unlocking the Power of Healthcare Datasets for Machine Learning
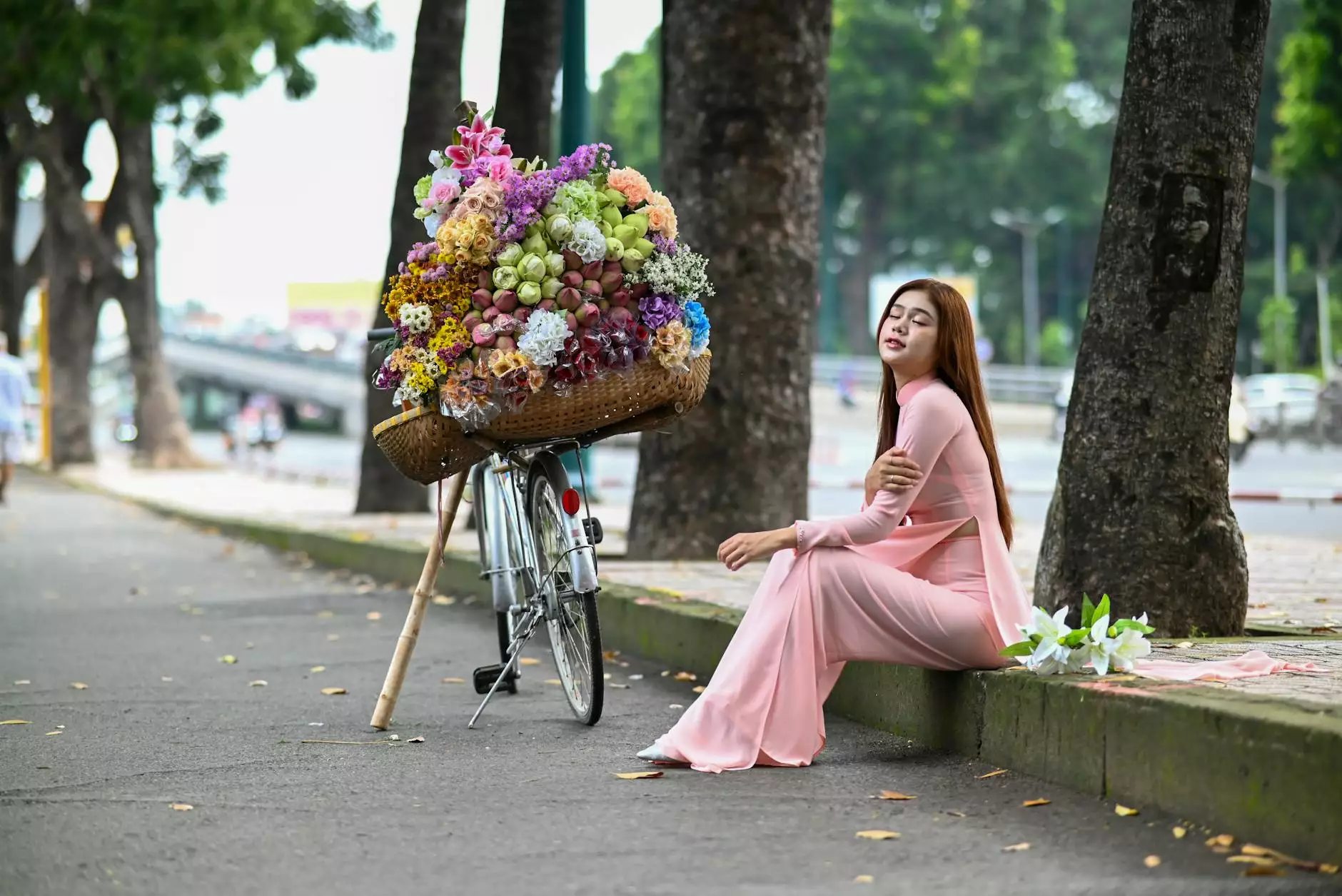
The field of healthcare has undergone a significant transformation in recent years, largely driven by advancements in technology. One of the most exciting developments is the use of healthcare datasets for machine learning, which continues to revolutionize patient care, medical research, and operational efficiency. By harnessing the power of machine learning algorithms on comprehensive datasets, healthcare providers can deliver better outcomes, personalize treatments, and streamline processes. This article delves deep into the world of healthcare datasets for machine learning, discussing their importance, applications, challenges, and future potential.
The Importance of Healthcare Datasets
Healthcare datasets are a treasure trove of information that can drive innovation and improve health outcomes. These datasets provide essential insights that can inform clinical decision-making, enhance research efforts, and support the development of predictive models. Here are some key reasons why healthcare datasets are invaluable:
- Informed Decision-Making: Healthcare professionals rely on data to make informed decisions regarding patient care.
- Understanding Population Health: Datasets help in analyzing trends, diseases, and the overall health of populations.
- Operational Efficiency: Streamlining processes through data can lead to cost savings and improved resource allocation.
- Drug Development: Data-driven insights speed up the process of developing new drugs and therapies.
Common Types of Healthcare Datasets
Healthcare datasets can come from a variety of sources and formats. Understanding these datasets is crucial for effectively applying machine learning techniques. Here are some prevalent types of healthcare datasets:
- Electronic Health Records (EHR): Comprehensive digital records of patient health information.
- Medical Imaging: Datasets from radiology, MRI, and CT scans for analysis and interpretation.
- Genomic Data: Information about an individual's genetic makeup that can help in personalized medicine.
- Clinical Trials Data: Data collected during clinical trials to evaluate new drugs and treatments.
- Wearable Device Data: Information from fitness trackers and health monitors that provide ongoing health insights.
Applications of Healthcare Datasets in Machine Learning
Healthcare datasets for machine learning have countless applications. Here are several key areas where these datasets play a crucial role:
1. Predictive Analytics
Machine learning models can predict disease outcomes based on historical patient data. For example, predicting the likelihood of hospital readmissions allows healthcare providers to intervene early and reduce risks.
2. Personalized Medicine
By analyzing genomic data alongside electronic health records, practitioners can tailor treatments to individual patients, considering their unique genetic profiles and health histories.
3. Medical Imaging Analysis
Machine learning algorithms can analyze medical images to detect anomalies (like tumors) with high accuracy, sometimes exceeding the capabilities of human radiologists.
4. Drug Discovery
Healthcare datasets fuel drug discovery processes by identifying patterns and relationships in how different treatments affect various patient demographics.
5. Operational Optimization
Hospitals can analyze operational data to streamline processes, reduce wait times, and optimize patient flow for better service delivery.
Challenges in Using Healthcare Datasets for Machine Learning
While the potential of healthcare datasets for machine learning is vast, several challenges must be addressed to maximize their effectiveness:
- Data Privacy and Security: Protecting sensitive patient information is paramount. Compliance with regulations such as HIPAA is crucial.
- Data Quality: Incomplete, inconsistent, or inaccurate data can lead to misleading results. Ensuring data integrity is essential.
- Integration of Diverse Data Sources: Combining datasets from various sources can be complex and may require sophisticated data management techniques.
- Bias in Machine Learning Models: If training datasets are not representative, it can lead to biased algorithms that perform poorly in real-world applications.
The Future of Healthcare Datasets and Machine Learning
The future of healthcare datasets and their application in machine learning is extraordinarily promising. As technology progresses, we can expect several key trends to shape the landscape:
1. Increased Data Collaboration
Healthcare organizations are increasingly collaborating to share data while adhering to regulations, leading to more comprehensive insights and better research outcomes.
2. Enhanced Real-Time Analytics
The integration of real-time data from wearable devices and mobile apps will allow healthcare providers to monitor patient health continuously, enabling proactive interventions.
3. AI-Driven Personalized Care
By leveraging vast datasets, artificial intelligence will drive even more personalized treatment plans that cater specifically to individual patient needs.
4. Standardization of Data Formats
Efforts to standardize data formats across various healthcare systems will facilitate easier data sharing and integration, optimizing the use of machine learning algorithms.
5. Empowered Patients
With healthcare datasets and machine learning models becoming more prevalent, patients will have access to personalized health insights that empower them to make informed decisions about their care.
How KeyMakr Can Help Leverage Healthcare Datasets
At KeyMakr, we specialize in Software Development solutions tailored to extract value from healthcare datasets for machine learning. Our team of experts can assist your organization in:
- Data Management: Designing systems that effectively manage and secure healthcare data.
- Machine Learning Solutions: Creating customized algorithms that derive valuable insights from your datasets.
- Regulatory Compliance: Ensuring that your data practices align with industry regulations to protect patient privacy.
- Integration Services: Developing seamless integration strategies for diverse data sources to maximize analytical capabilities.
Conclusion
Healthcare datasets for machine learning hold the key to unlocking unprecedented advancements in patient care, medical research, and healthcare operations. By understanding and leveraging these datasets, organizations can not only enhance their services but also contribute to a healthcare system that is more efficient, personalized, and capable of meeting the challenges of today and the future. At KeyMakr, we are committed to helping you harness the full potential of your healthcare data, paving the way for innovations that can transform lives.